Retail Pricing: Should I Price for Value or for Profit?
If this question does not make sense to you, read on.
What is value-based pricing?
In retail, many consider value pricing—i.e., pricing a product based on its value to the customer—the gold standard.
In most cases, the value of a product is determined for relevant customer segments—for example, by looking at buyer personas. Consider the following ski jacket example:
A retailer offers four models marketed to previously identified customer segments. The jackets are differentiated by two value drivers: filling (down and synthetic) and water column (high and low, i.e., above or below 15,000 mm).
Figure 1: Example buyer persona (low budget)
By applying value pricing and insights from identified buyer personas (see Figure 1), the retailer determined the average willingness to pay (WTP) for the base jacket (synthetic and low water column) to be €250.
Further, the average WTP for the down filling vs. the synthetic filling is an additional €50, and the WTP for the high water column (>15,000mm) vs. the low water column is also €50.
Figure 2 shows the value price model and the resulting prices of the four jackets. The Blue model targets premium customers; the Red Model targets skiers who want to stay warm but are less concerned about getting wet; the Black model targets sportive skiers who need to stay dry; and the Gray model is the budget model.
Figure 2: Ski jacket offer based on value pricing
This retail pricing approach is straightforward and applied by thousands of retailers worldwide. However, it almost always leaves money on the table because, besides ignoring costs, it more crucially ignores customers’ tradeoff decisions between offers and how a portfolio can be optimized to consider these effects.
For example, if the Blue jacket was €329, it might pull significant demand from the Red and Black jackets (and current non-buyers), leading to a higher total profit.
Define shoppers' willingness to pay
To evaluate such scenarios, let us look deeper into what is happening underneath the value pricing hood.
The simplification—manifested in buyer personas and customer segments—of treating all customers (in a segment) as their average helps marketers better conceptualize the market, but it comes at a cost.
We know from empirical customer preference studies that customers do not share a common willingness to pay (WTP) for a product. Rather, some would pay more and some less than the average.
The result is a distribution like the one shown in Figure 3. It differs sharply from the (implicit) assumption of equal preferences made by value pricing.
Taking advantage of variations in customers’ WTP requires different tools. For example, if a marketer is only concerned with one product and the demand function is known (e.g., produced by the blue distribution in Figure 3), straightforward price elasticity evaluations provide a good tool for profit optimization.
Figure 3: WTP for a product (for a customer segment)
In a multi-product case, optimization is more difficult.
Figure 4 illustrates the ski jacket case reduced to just the two down jacket models. Each potential customer has a WTP for each of the two jackets. Someone with a strong preference for staying dry will be willing to pay significantly more for the Blue model than the Red one.
Some potential customers will not buy either jacket at the current prices of €350 and €300, respectively (quadrant I). Some would only buy the Blue model (II) or the Red model (IV). Some customers would buy either one and must choose given the two jackets’ prices and their personal preferences for each model (III).
Marketers must examine relevant price variations and evaluate customers’ expected purchases to maximize profit. For more than two products, this becomes difficult to do with paper and pencil—or Excel.
That’s why we built Buynomics.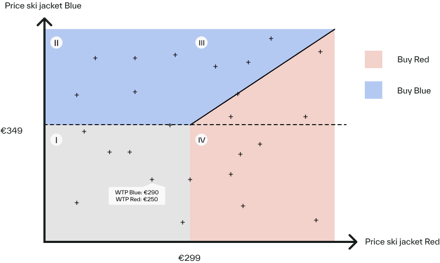
Figure 4: Customers' willingness to pay for down jackets
Pricing with Buynomics
We created our Virtual Shopper AI for this ski jacket case study based on customers’ preferences from a similar retail project. Virtual shoppers share the demographics, preferences, and WTP for products of their real-life counterparts and, as a group, make the same purchase decisions when faced with the same offers. This makes them an ideal tool for analyzing the effects of price changes on the demand for different products.
Figure 5 compares the value pricing solution to the profit optimum identified by Buynomics. The Buynomics method leads to a profit improvement of 8% against value prices*.
In this case study, the retailers reduced the price spread between the top (Blue) and the bottom (Gray) models to facilitate better upselling, which was the main profit lever.
Note that the Gray jacket has very low sales (13 units) because the price is very close to that of the Red and Black jackets. In an optimal situation, the price of the Gray jacket is reduced by €20, sales increase to 1038 units, and the profit loss is limited.
The pricing manager should analyze the effects of price changes on sales, revenue, and profit and then evaluate the price structure. For example, how does a low entry price affect profits? How does a close competitor affect sales?
Buynomics' platform can study both questions.
Figure 5: Comparison of value prices and profit optimum determined by Buynomics
Please note that the proposed reduction in the portfolio's price spread is specific to this example and will not always result from price optimization. However, we frequently find that standard value pricing methods lead to a too-wide price spread in a portfolio and profits significantly below the optimum.
Take a look at the Buynomics product to see for yourself how sales react to price changes.
In this example, the virtual shoppers were constructed from historical sales data, validated by the client’s marketing and sales teams, and confirmed by subsequent sales.
Buynomics can train its virtual shoppers to perform according to various behavioral traits, such as susceptibility to price thresholds or a preference for a middle option, wherever relevant. The methods can be used to develop and price new products and improve existing prices.
Further, because the method produces reliable predictions of future sales volumes depending on their prices, Buynomics is also capable of aiding sales forecasting.
Want to know more? Schedule a call with us today!
* In the actual retail case, the profit increase was also 8%, and sales volumes, prices, and costs were proportional to those shown in this case study.

April 25, 2023